AI Risks and How to Start Now with AI – AI in Retail Part 3
Retail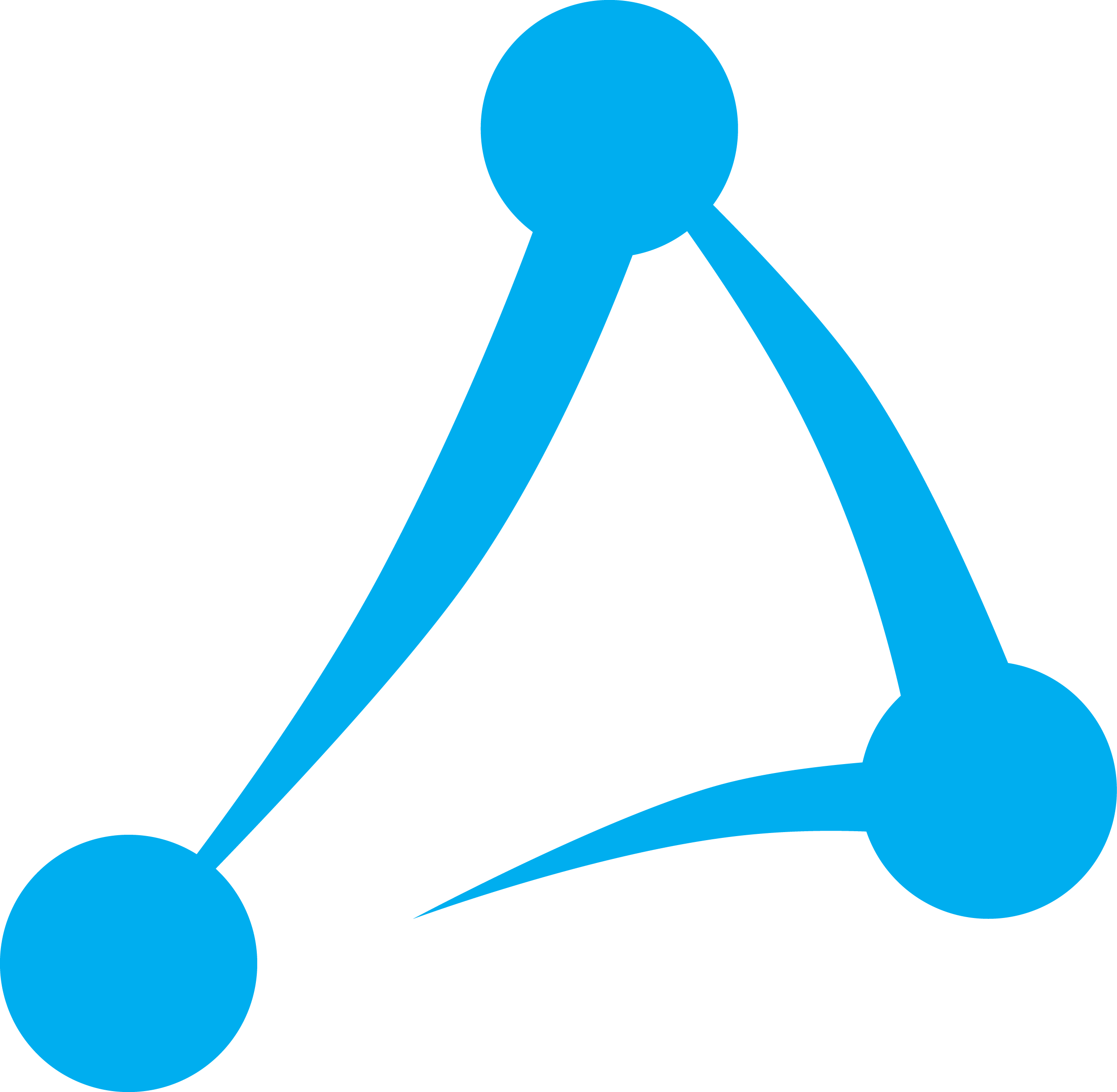
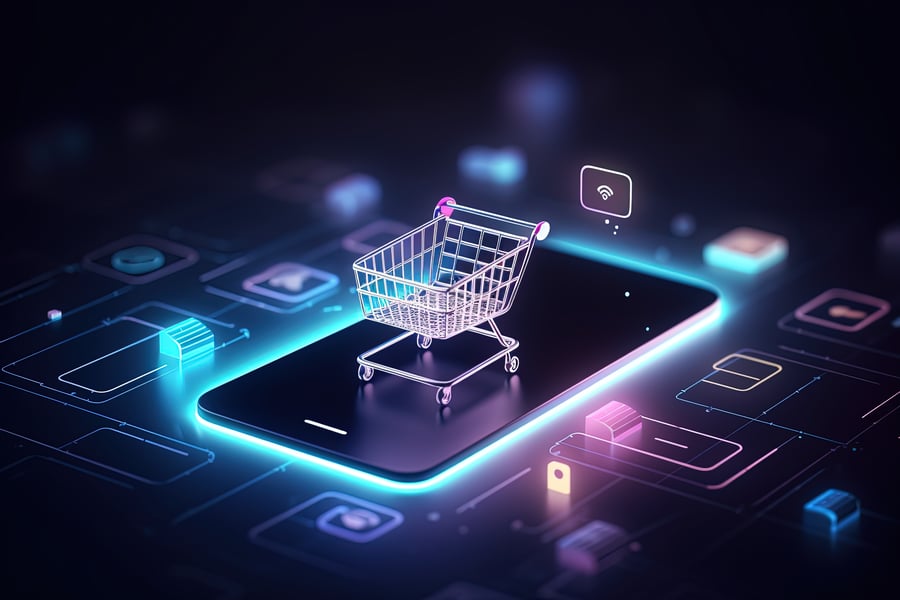
Since the release of OpenAI’s ChatGPT at the end of 2022, there’s been a big question on the mind of retail leaders: how will the current breakthroughs in artificial intelligence (AI) technologies impact retailers and their businesses in the years ahead?
To answer that, we’ve released this 3-part series inspired by IHL Group’s original new Agilence-sponsored report, Retail’s AI Revolution.
In Part 1 of this AI in Retail series, we defined the main subfields of AI, how these areas apply to retail, and the expected economic impact on retailers over the next decade.
In Part 2, we explored the opportunities for retailers that AI brings, including sales and revenue growth opportunities, supply chains and gross margin impact, and how AI will reduce operating expenses.
But with great opportunity also comes risk. That’s why in Part 3, we wrap up this series by exploring some of the risks for retailers with adopting new AI technologies, the prerequisites (*cough* data quality) for reaping their benefits, as well as getting started with the solutions that are ready-to-go today.
The Importance of Data Quality
When it comes to both AI/ML and Generative AI (GAI), data quality is critically important. Data quality directly impacts the performance, reliability, and accuracy of AI models. High-quality data enables AI models to make better predictions and produce more reliable outputs. The more accurate, tagged, and abundant (narrowed to your specific use case) the data, the better the results.
The old adage “garbage in, garbage out” is of the utmost importance in traditional AI/ML. This means that if the input data to a system such as an AI model or algorithm is of poor quality, inaccurate, or irrelevant, the system’s output will be as well. This is particularly significant for AI as AI models rely heavily on the data used for training. If you start deploying AI/ML on incorrect data, you simply get wrong decisions much faster.
A study conducted by Refiniv in 2019, revealed that the biggest barrier to the deployment and adoption of AI/ML is bad data quality. According to the survey, 66% of respondents said that poor data quality affects their ability to deploy AI/ML technologies. The report also suggests that three of the four challenges of working with new data in ML models are related to data quality, including inaccurate information about the history, coverage, and population of data; identifying incomplete or corrupt records; and cleaning and managing data. One of the biggest challenges data scientists face is finding good quality data, as they have to spend 80-90 percent of their time cleaning and normalizing bad data.
Implementing a data quality practice
It’s easy to see how poor data quality can cause your AI models to be detrimental to your business. There are few shortcuts to clean data. Companies can use third-party services or even other AI tools to help assist, but you cannot skip the cleaning step and still expect superior results from AI/ML. Here are some steps to improve your data quality now and going forward into the future:
- Implement a data governance framework. Ensuring that all data points follow the same standards and eliminating duplicate or conflicting data is complex. A robust data governance framework should be in place to define data quality standards, processes, and roles.
- Get help from outside software and service providers. AI algorithms rely on labeled data for training, but manual labeling is both time-consuming and prone to errors. Data labeling is one of the biggest challenges for AI adoption, and you will need to either outsource this process or build an in-house team. Make sure to leverage data quality tools that can help automate data cleansing, validation, and monitoring processes, ensuring that AI models have access to high-quality data consistently.
- Ensure your data is stored securely. Maintaining data quality also means protecting it from unauthorized access and potential corruption. Ensuring secure and robust data storage is critical for organizations.
- Build a data quality team. Having a dedicated team responsible for data quality ensures continuous monitoring and improvement of data-related processes. The team can educate and train other employees on the importance of data quality, collaborate with your data providers, and continuously monitor data quality metrics.
Generative AI accuracy, bias, and copyright issues
If you’ve been following the hype around ChatGPT or using GAI tools powered by Large Language Models (LLMs), you may have encountered the issue of “hallucinations,” where the model outputs factually wrong or otherwise problematic text. This is a real issue because of the way in which LLMs work – at this point, they are not pulling information from the Internet but “predicting” text and have no mechanism to check the accuracy of outputs. This issue can be ameliorated somewhat by giving LLMs specific goals and relevant context and having a human double check factual claims from GAI applications.
Along with these can be risks of biased outputs, as well as issues with content originality and copyright issues. Copyright issues are still being hashed out, so when using GAI to create public-facing content, it’s a good idea to be prudent when publishing.
Because of the nature of Generative AI use cases for retail, these issues are less severe than with AI/ML. Whereas AI/ML systems need to get to an answer and convert it to specific actions or a range of values from which a human can choose, Generative AI tools will be a supplement for administrators, marketers, and other back office workers, who can use the outputs with discretion, but will be able to become much more productive with them.
Job displacement
Any discussion about AI would be incomplete without addressing the concerns surrounding job displacement.
The World Economic Forum predicts that nearly a quarter of all jobs will change as a result of AI over the next 5 years, with AI resulting in “significant labor-market disruption.” While it’s exactly what the long-term effects of AI on the retail labor market will be, it seems to provide an opportunity for retailers facing major staffing challenges.
AI will impact positions all along the supply chain. With a global shortage of retail workers relative to the existing number of stores, large retailers are already experimenting with AI and robotic automation to augment in-store workers. Companies such as Amazon and DHL have already been experimenting with robots for supply chain tasks for years. If you read Part 2 of this series, you will see the massive impact AI is having and will continue to have on non-frontline positions ranging from administration to marketing to customer service.
And yet, retailers continue to face a skills and labor shortage. While those who resist learning and utilizing AI will likely replace those who embrace it, widespread layoffs solely due to AI are unlikely within the next 7-10 years. Throughout history, from the printing press to barcodes, the internet, and mobility, technological advancements have consistently transformed retail. The industry has shown resilience and adaptability, with skills evolving alongside these changes. We anticipate no less in this era. Employees who demonstrate willingness to adapt and grow will experience significant productivity gains, leading to a reduction in repetitive and mundane tasks.
Security
Finally, AI will have a huge impact on security for retailers. These technologies not only amplify the abilities and efficiencies of retailers but also of bad actors, hackers, and scammers. AI enhancements are crucial for retailers to adopt in their network to protect all endpoints, as well as to enhance physical security.
The retail industry has witnessed a swift proliferation of customer-facing interface points in recent years, largely driven by the changes brought about by the pandemic and labor shortages. This expansion encompasses a wide range of innovations, such as self-checkouts, Scan and Go systems, the widespread adoption of electronic shelf labels (ESLs), as well as the introduction of various kiosks and other devices. At the same time, retailers have greatly increased the number of IoT solutions, ranging anywhere from cameras to RFID and Computer Vision to freezers, fryers, robots, logistics tools and more. All of this has led to an exponential increase in the size of the threat vector.
The CIO’s challenge is being consistent in the process and procedures for each new device that connects but still being responsive to the demands of the business units. Each provides a different attack surface so CIOs must be consistent in approach but diverse in the manner each is secured. Often, outside departments are driving IT purchases faster than IT can keep up, opening up possible security holes with potentially disastrous consequences as the number of attack surfaces exponentially increases. Generative AI has provided malicious actors with an unprecedented arsenal of tools, enabling them to exponentially amplify their attacks and exacerbate problems.
Using outside Generative AI tools can also expose retailers to data security risks, as data fed into these tools is saved by outside companies and can even be used to train future models. According to the results of a recent Salesforce survey, 71% of 500 senior IT leaders postulate that generative AI is likely to “introduce new security risks to data.” One of these risks is employees sharing confidential information with GAI tools. Research by Cyberhaven found that 11% of the data that employees paste into ChatGPT is confidential, and 4% of employees have pasted sensitive data into it.
The good news is that the advances in Generative AI can also be used by retail IT and security teams to better protect networks as well as assist in providing better physical security for associates and customers. Security should be the first thing that CIOs and CISOs should be looking at as they look to take advantage of AI technology.
Getting started with AI solutions in retail
Due to previous investments in data cleansing and other pressing needs, there are many solutions that are already bringing returns with the help of AI tools. In general, they are solutions that reduce the reliance of labor at the store and supply chain levels, those that increase margins on digital orders (particularly those that have a store-fulfillment component), those that improve on-shelf availability, those that impact pricing and optimization, and those that impact loss prevention.
Some examples include:
- AI in Self-Checkout
- Store Performance Tools
- Merchandising/Supply Chain/Category Management
- Pricing
- Loss Prevention
To learn about these examples and read the complete findings from IHL, download the report for free today.
Related Articles
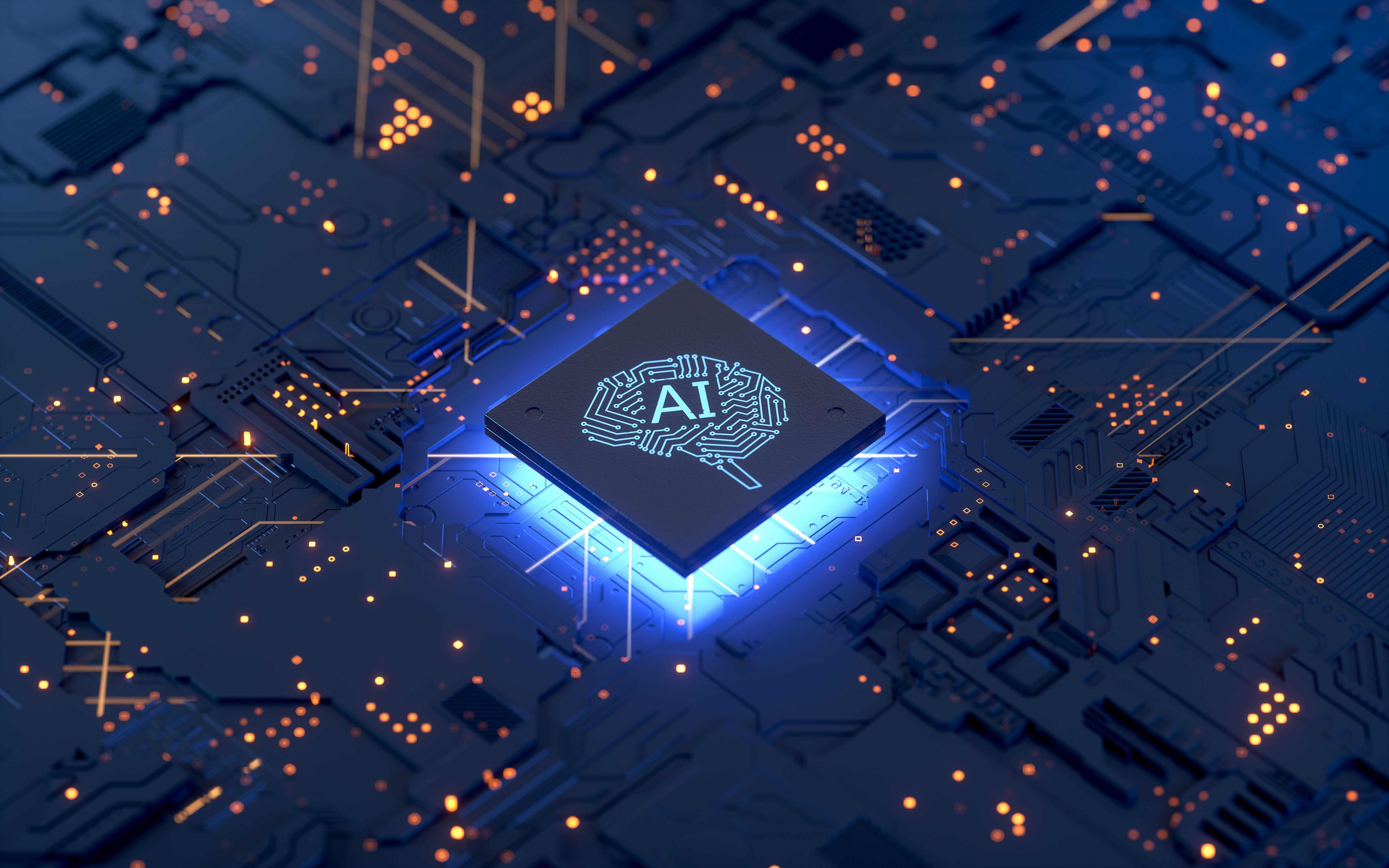
AI in Retail Part 1: What is AI and How Will It Impact Retail in the 2020s?
Since the release of OpenAI’s ChatGPT in late 2022, artificial intelligence (AI) has become one of the hottest topics for bus...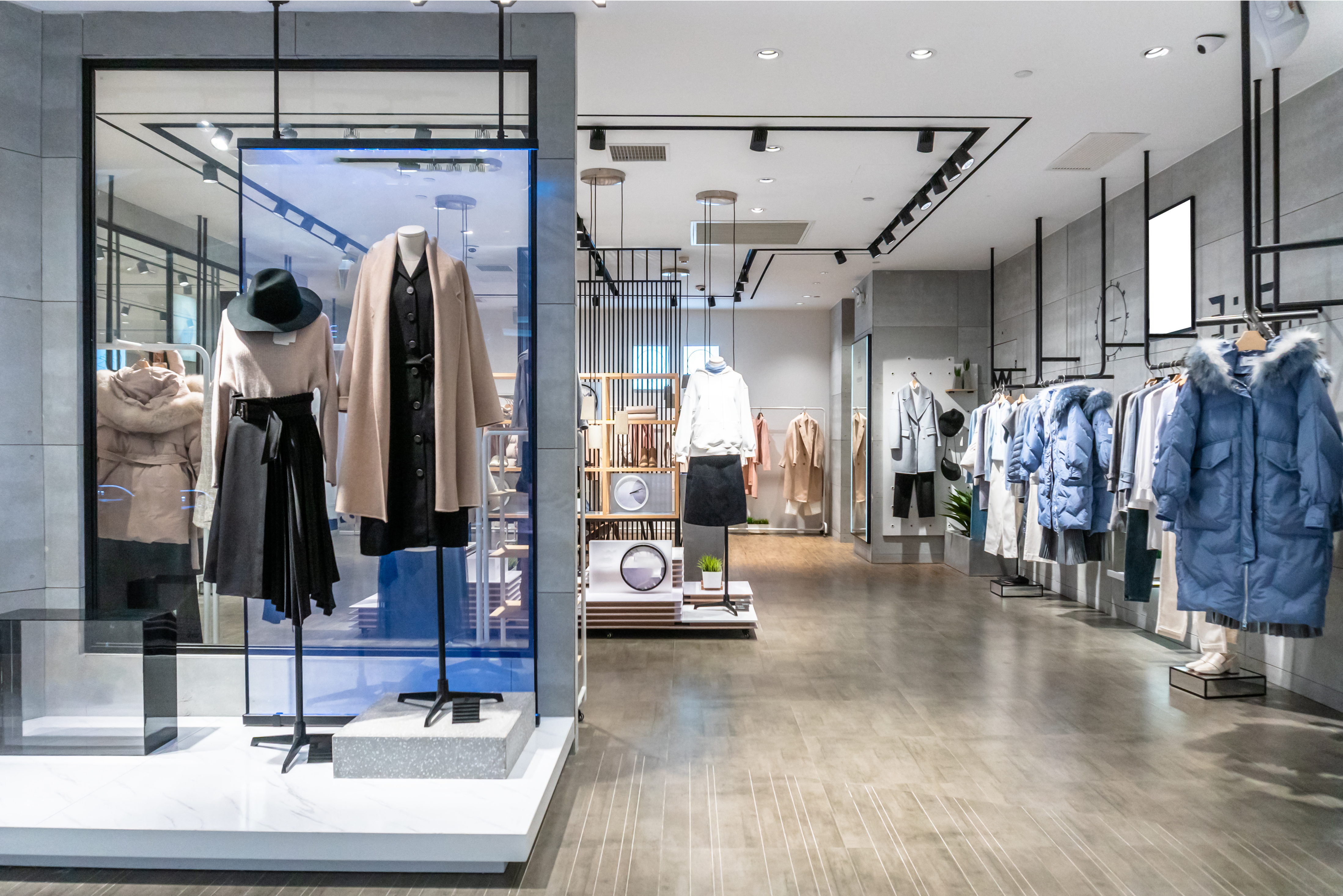
Webinar Recap - Retail's Digital Journey: How Winning Retailers Are Controlling Shrink and Thriving
In our recent webinar, Retail’s Digital Journey: How Winning Retailers are Controlling Shrink and Thriving, Greg Buzek of IHL...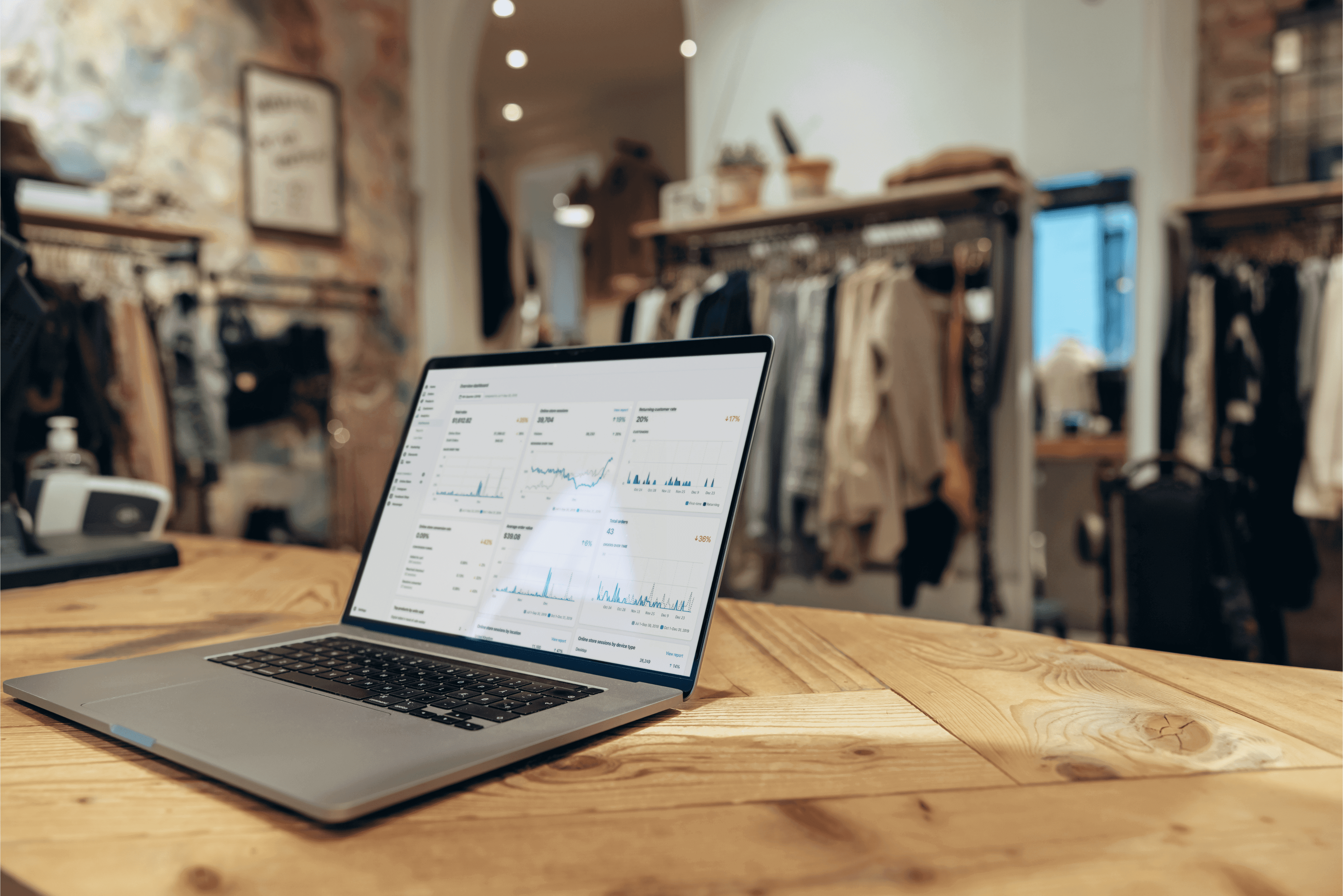
Where Winning Retailers are Spending
As retailers regain momentum in a post-COVID environment, winning retailers now take a hard look at the bottom line. What inv...Subscribe to our blog
Receive free educational resources like exclusive reports, webinars, and industry thought leadership articles straight to your inbox.