6 Ways to Ensure your Data Analytics Efforts Fail
General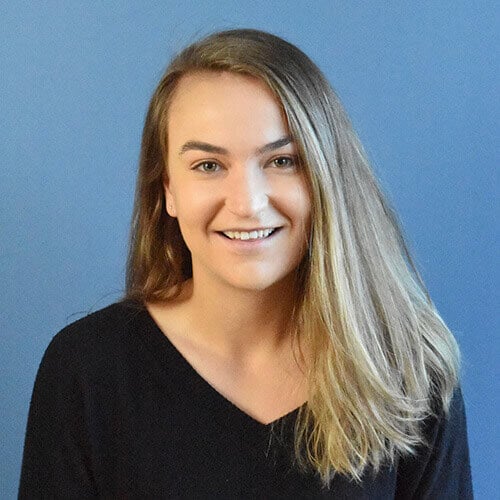
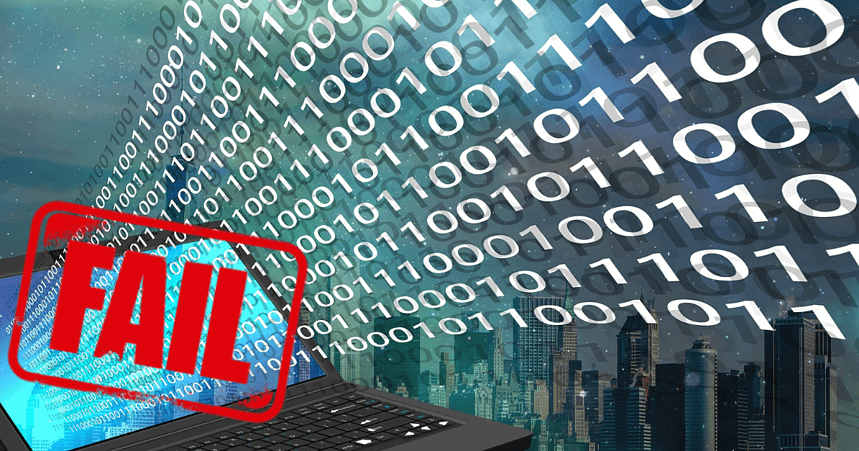
In late 2017, Gartner revealed that about 60% of big data analytics and business intelligence projects fail to move past the preliminary piloting and experimentation phases and will ultimately be abandoned. Shortly after, one of their industry analysts revealed that they were too conservative in their estimate, and the real metric of failure is closer to 85%. Another study from McKinsey found that US retailers have captured only 30-40% of the potential value of their data. Lack of analytical talent, unsupportive leaders, failure to prove utility, and siloed data within companies are most often to blame for the value left on the table. Getting started with advanced analytics is as much about changing mindsets and culture as it is about acquiring tools and skills. However, with such a high failure rate, the majority of organizations are attempting to utilize data analytics without the right approach, skills, tactics, and tools.
Here are 6 sure-fire ways to ensure that your BI and Data Analytics efforts fail:
1. Jump in Without Knowing what you’re Looking For
A sure recipe for failure is lacking focus when launching any analytics effort. Perhaps the most common reason for failure, many companies begin data analytics projects with no idea what they’re looking for in their data. There is an all too common misconception that systems will somehow discover what’s interesting in the data and deliver it to users on a golden platter. As technologies like Machine Learning become more and more common, this isn’t beyond the realm of possibility. However, if users feed Machine Learning and Artificial Intelligence technologies the wrong search criteria, they’re still likely to get false positives or inaccurate returns on what they’re truly trying to target. Ultimately, data teams will always be most successful when they are focused on a prioritized set of outcomes.
2. Be a Data Divider, Not a Data Champion
Enterprises have long struggled with data silos or repositories of fixed data, each belonging to a department or business function that is isolated from the rest of the organization. These silos have made it difficult to manage, analyze, and activate data which hampers the identification of valuable opportunities for enterprise-wide improvement. Often, the task of breaking down these data silos falls to a data champion, whose goal is to advocate for the importance of collecting and leveraging data, supporting the use of data analytics in decision-making, and actively promoting data analytics best practices throughout the organization.
3. Embrace Dirty Data
“Garbage in, garbage out.” If data is not accurate, up to date, well organized, and easily understood, the value of data analytics drops drastically. Moreover, it only takes one or two people saying, “I don’t trust the data,” to invalidate a report. When reports become useless it can tank a whole project. High quality insights from data analysis requires clean, high quality data. Organizations must adopt a strong validation process that focuses on enabling access to all the data needed to answer queries without sacrificing data integrity.
4. Forgo Organizational Buy-In of Analytics Initiatives
As with any other major enterprise-wide cultural shift, forgoing buy-in from the entire organization, from executives to end-users, can be a detriment to success. Creating a data-driven future requires sustained effort, often over multiple years. To ensure follow-through, C-suite and business leaders must take an active role to champion data analytics initiatives and remain committed to their success. Without executive or stakeholder buy-in, analytics efforts will have a difficult time obtaining proper funding or stakeholder backing. But without end-user buy-in, data will not be utilized correctly, and the potential value will be lost. This is why one of the the greatest obstacles to realizing big data success is an internal culture issue.
5. Focus on One or Two Departments Rather than a Company-Wide Effort
Data Analytics projects are often driven by a specific goal or project for an internal department. Unfortunately, these successes rarely extend beyond the initial departmental goal or project. Once the goal is reached, the organization loses further benefit because it’s not extended beyond the initial scope. For this success to grow and to create a truly data-driven enterprise, leaders must take a holistic view of data analytics, driving momentum from the small successes to even bigger wins.
6. Rely on Generic Systems or Build your own from Scratch
Another common reason for data analytics failure is an all or nothing approach to infrastructure in terms of either relying on a “one-size-fits-all solution” or building a custom solution in-house. Either of these can jeopardize the mission of your analytics team by wasting time and efforts on tasks other than developing better analytics. Generic solutions often require extensive customizations and configurations to adhere to industry-specific processes, best practices, and regulatory requirements. Similarly, custom solutions must be built from scratch to a company’s unique requirements and often require extensive ongoing maintenance, limiting flexibility and enterprise agility. Instead of relying on a generic system or building your own, utilize a specialized, vertically-focused business intelligence or data analytics system to maximize enterprise-wide impact and value.
We at Agilence have developed vertical-focused business intelligence and data analytics solutions for the retail, restaurant, grocery, c-store, and pharmacy industries to provide enterprise-wide insights and improve decision-making. A solution like 20/20 Data Analytics can consolidate various data sets, identify patterns or events that warrant attention, use automated alerts to inform appropriate personnel of any anomalies, and finally, utilize various visualization methods to deliver easy-to-understand, actionable insights.
Learn more about how to make your data analytics efforts a success in my on-demand webinar, "Democratize your Data: Overcoming Internal Information Silos!"
Related Articles
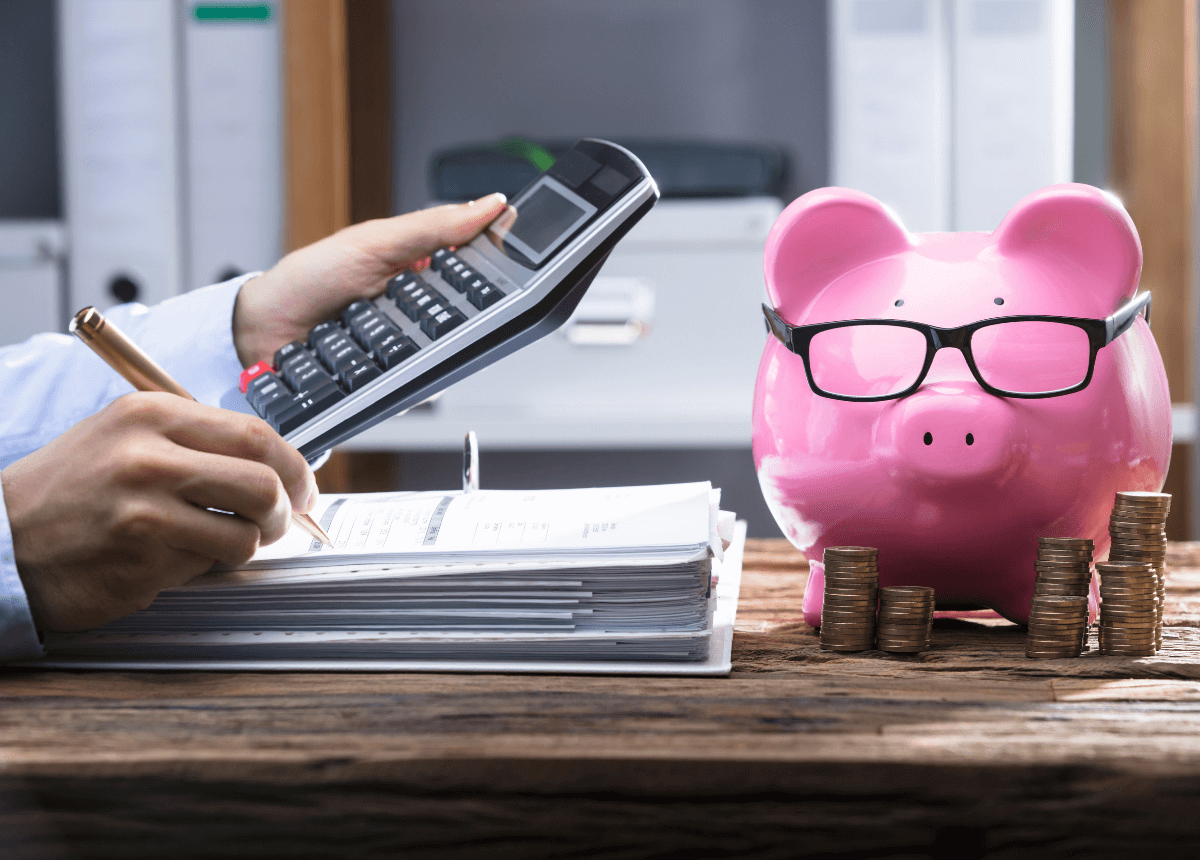
Calculating Total Cost of Ownership for Data Analytics
Most companies are incredibly attentive to the hard costs of a data analytics & reporting investment, i.e., per-user lice...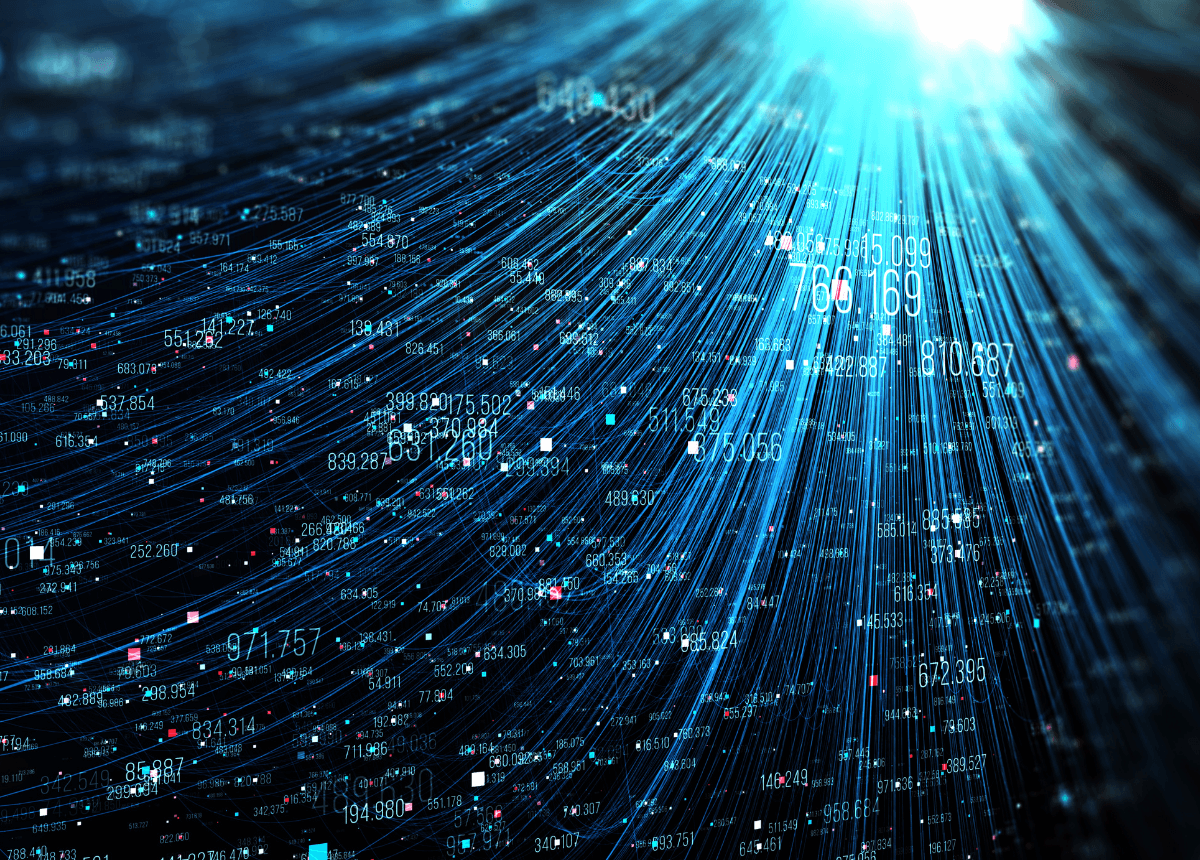
Why Loss Prevention Leaders Must Become Analytics Evangelists
As retailers bounce back from the pandemic, they’re simultaneously finding innovative ways to serve customers while controlli...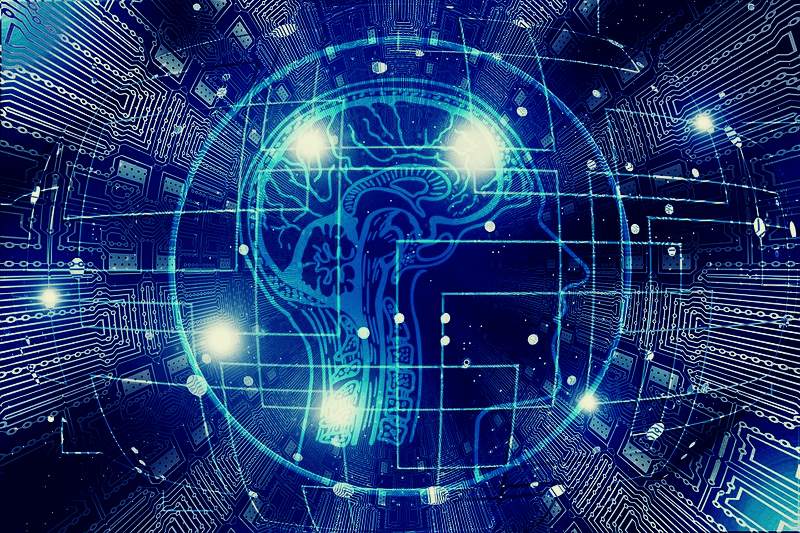
The Psychology Behind Business Dashboards
Within the past decade, dashboards have become an increasingly popular way for businesses to internally display data. But, wh...Subscribe to our blog
Receive free educational resources like exclusive reports, webinars, and industry thought leadership articles straight to your inbox.